The relationship of economic sentiment and GDP growth in Russia in light of the Covid-19 crisis
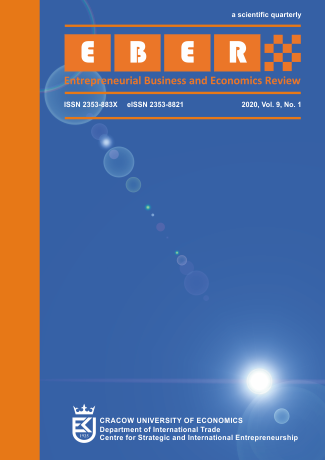
Abstract
Objective: The objective of the article is to prove the empirical and predictive value of the aggregate opinions of businesses and households for expanding cyclical macroeconomic data in Russia, especially during the coronavirus shocks.
Research Design & Methods: We use qualitative information from surveys that cover about 24 000 organisations and 5100 households in all Russian regions. The total economic sentiment indicator (TESI) combines information on 18 survey-based indicators. Cross-correlation analysis, Hodrick-Prescott filtering, and a vector autoregressive (VAR) model with dummy variables are used as the research methods.
Findings: The study confirms an almost synchronous cyclic conformity of the gross domestic product (GDP) growth and TESI dynamics for the period of 1998-2020. Probable GDP growth until the end of 2021 is estimated based on the expected impulses in the TESI dynamics, including those due to the sudden impact of the coronavirus.
Implications & Recommendations: Assessments of business and household activity are reliable and available much earlier than quantitative statistics on GDP growth. Therefore, we advise to use them as an early warning system about economic growth and take them into account in policymaking.
Contribution & Value Added: We are the first to confirm the effectiveness and reliability of TESI as a leading indicator of GDP growth in Russia, using data from large-scale business surveys and with a focus on crisis shocks.
Keywords
business and consumer surveys; economic sentiment indicator; composite indicators; economic growth; GDP growth; growth cycles
Author Biography
Liudmila Kitrar
Candidate of Sciences (PhD) in Mathematical and Instrumental Methods in Economics; Deputy Director of the Centre for Business Tendency Studies, Higher School of Economics, Moscow, Russian Federation; member of the Russian Association of Statisticians; UNIDO international expert. Her research interests include: indicator approach to measuring economic dynamics; methods of quantification, analysis and visualization of nonparametric statistics; econometric methods of decomposition of unobservable variables in economic dynamics; entrepreneurial expectations; VAR models and statistical filtering in the practice of business tendency monitoring.
Tamara Lipkind
Leading expert of the Centre for Business Tendency Studies, Higher School of Economics, Moscow, Russian Federation. Her research interests include: composite indicators of business cycles; business and consumer surveys; sectoral and regional comparative analysis of economic sentiment and business climate; quantification and visualisation of nonparametric statistics.
Correspondence to: Tamara Lipkind, National Research University Higher School of Economics, Institute for Statistical Studies and Economics of Knowledge, Centre for Business Tendency Studies, Slavyanskaya Sq., 4/2, Moscow, 101000, Russian Federation, e-mail: tlipkind@hse.ru
References
- Angelini, E., Camba-Méndez, G., Giannone, D., Rünstler, G., & Reichlin, L. (2008). Short-term forecast of euro area GDP growth. ECB Working Paper, 949. Retrieved from https://ssrn.com/abstract=1275821 on August 1, 2020.
- Astolfi, R., Gamba, M., Guidetti, E., & Pionnier, P.A. (2016). The Use of Short-Term Indicators and Survey Data for Predicting Turning Points in Economic Activity: A Performance Analysis of the OECD System of CLIs during the Great Recession. OECD Statistics Working Papers, 2016/08. https://doi.org/10.1787/5jlz4gs2pkhf-en
- Bachmann, R., Elstner, S., & Sims, E.R. (2013). Uncertainty and economic activity: Evidence from business survey data. American Economic Journal: Macroeconomics, 5(2), 217-249. https://doi.org/10.1257/mac.5.2.217
- Banbura, M., & Runstler, G. (2007). A look into the factor model black box – publication lags and the role of hard and soft data in forecasting GDP. ECB Working Paper, 751. Retrieved from https://ssrn.com/abstract=984265 on August 1, 2020.
- Banbura, M., Giannone, D., & Reichlin, L. (2010). Large Bayesian VARs. Journal of Applied Econometrics, 25(1), 71-92. https://doi.org/10.1002/jae.1137
- Banbura, M., Giannone, D., & Lenza, M. (2014). Conditional forecasts and scenario analysis with vector au-toregressions for large cross-sections. ECARES Working Paper, 15.
- Basselier, R., de Antonio Liedo, D., & Langenus, G. (2017). Nowcasting real economic activity in the euro area: Assessing the impact of qualitative surveys. National Bank of Belgium Working Paper, 331. Re-trieved from https://www.nbb.be/doc/ts/publications/wp/wp331en.pdf on August 2, 2020.
- Bernanke, B., Boivin, J., & Eliasz, P. (2005). Measuring the effects of monetary policy: A factor-augmented vector autoregressive (FAVAR) approach. The Quarterly Journal of Economics, 12, 387-422. https://doi.org/10.3386/w10220
- Biau, O., & D'Elia, A. (2011). Is There a Decoupling Between Soft and Hard Data? The Relationship Between GDP Growth and the ESI. In Proc. of the Fifth Joint EU-OECD Workshop on International Developments in Business and Consumer Tendency Surveys, Brussels. 2011, 17-18 November.
- Bloom, N. (2014). Fluctuations in Uncertainty. Journal of Economic Perspectives, 28(2), 153-176. https://doi.org/10.1257/jep.28.2.153
- Bonadio, B., Huo, Zh., Levchenko, A., & Pandalai-Nayar, N. (2020). Global Supply Chains in the Pandemic. CEPR Discussion Paper, DP14766. Retrieved from https://ssrn.com/abstract=3603998 on August 1, 2020.
- Bry, G., & Boschan, Ch. (1971). Cyclical Analysis of Time Series: Selected Procedures and Computer Programs. New York: National Bureau of Economic Research.
- Cesaroni, T. (2011). The Cyclical Behavior of the Italian Business Survey Data. Empirical Economics, 41(3), 747-768. https://doi.org/10.1007/s00181-010-0390-7
- Cesaroni, T., & Iezzi, S. (2017). The Predictive Content of Business Survey Indicators: Evidence from SIGE. Journal of Business Cycle Research, 13(1), 75-104. https://doi.org/10.1007/s41549-017-0015-8
- D´Amato, L., Garegnani, L., & Blanco, E. (2015). GDP Nowcasting: Assessing business cycle conditions in Ar-gentina. BCRA Working Paper Series, 2015/69. Retrieved from https://www.bcra.gob.ar/Pdfs/Investigaciones/WP_69_2015%20i.pdf on August, 01, 2020.
- Darracq Paries, M., & Maurin, L. (2008). The role of country-specific trade and survey data in forecasting euro area manufacturing production: perspective from large panel factor models. European Central Bank Working Paper, 894. Retrieved from https://ssrn.com/abstract=1120700 on August 1, 2020.
- De Mol, C., Giannone, D., & Reichlin, L. (2008). Forecasting using a large number of predictors: Is Bayesian shrinkage a valid alternative to principal components?. Journal of Econometrics, 146(2), 318-328.
- Drechsel, K., & Maurin, L. (2011). Flow of Conjuntural Information and Forecast of Euro Area Economic Activ-ity. Journal of Forecasting, 30(3), 336-354. https://doi.org/10.1002/for.1177
- Dubovskiy, D., Kofanov, D., & Sosunov, K. (2015). Datirovka rossijskogo biznes-cikla Dating of the Russian Business Cycle. HSE Economic Journal, 19(4), 554-575. (In Russian).
- European Commission (2014). What do survey data tell us about future price developments? (European Busi-ness Cycle Indicators – 2nd Quarter 2014, Publications Office of the European Union). Retrieved from https://ec.europa.eu/economy_finance/publications/cycle_indicators/2014/pdf/ebci_2_en.pdf on 23 Ju-ly, 2020.
- European Commission (2016). ‘New Normal’? – The Impact of the Financial Crisis on Business and Consumer Survey Data (European Business Cycle Indicators – 3rd Quarter 2016, Publications Office of the European Union). https://doi.org/10.2765/600396
- European Commission (2017). ESI and Other BCS Indicators vs PMI – Properties and Empirical Performance (European Business Cycle Indicators – 2nd Quarter 2017, Publications Office of the European Union). https://doi.org/10.2765/283995
- European Commission (2018). Nowcasting euro area GDP growth with Mixed Frequency Models (European Business Cycle Indicators – 1st Quarter 2018, Publications Office of the European Union). https://doi.org/10.2765/17446
- European Commission (2019). Recent Development in Survey Indicators (European Business Cycle Indicators - 2nd Quarter 2019, Publications Office of the European Union). https://doi.org/10.2765/870948
- European Commission (2020). The Joint Harmonised EU Programme of Business and Consumer Surveys. User Guide. Retrieved from https://ec.europa.eu/info/sites/info/files/bcs_user_guide_2020_02_en.pdf on August 20, 2020.
- Fernandes, N. (2020). Economic Effects of Coronavirus Outbreak (COVID-19) on the World Economy. IESE Business School Working Paper, WP-1240-E. https://doi.org/10.2139/ssrn.3557504
- Galli, A., Hepenstrick, C., & Scheufele, R. (2019). Mixed-Frequency Models for Tracking Short-Term Economic Developments in Switzerland. International Journal of Central Banking, 58, 151-178. Retrieved from https://www.ijcb.org/journal/ijcb19q2a5.htm on July 25, 2020.
- Gayer, С. (2008). Report: The Economic Climate Tracer. A Tool to Visualise the Cyclical Stance of the Economy Using Survey Data (European Commission). Retrieved from http://ec.europa.eu/economy_finance/db_indicators/surveys/documents/studies/economic_climate_tracer_en.pdf on July 25, 2020.
- Gayer, C., & Marc, B. (2018). A ‘New Modesty’? Level Shifts in Survey Data and the Decreasing Trend of ‘Nor-mal’ Growth. (European Economy Discussion Paper, 083). https://doi.org/10.2765/067112
- Giannone, D., Lenza, M., & Primiceri, G.E. (2012). Prior Selection for Vector Autoregressions. ECB Working Paper Series, 1494. Retrieved from https://ssrn.com/abstract=2176133 on August 1, 2020.
- Girardi, A. (2014). Expectations and macroeconomic fluctuations in the euro area. Economics Letters, 125(2), 315-318. https://doi.org/10.1016/j.econlet.2014.09.031
- Girardi, A., Gayer, C., & Reuter, A. (2015). The Role of Survey Data in Nowcasting Euro Area GDP Growth. Journal of Forecasting, 35(5), 400-418. Retrieved from https://ideas.repec.org/p/euf/ecopap/0538.html on July 25, 2020.
- Gollier, C., & Straub, S. (2020). The Economics of Coronavirus: Some Insights. Toulouse School of Economics: Public Policy. Retrieved from https://www.tse-fr.eu/economics-coronavirus-some-insights on November 20, 2020.
- Granville, B., & Mallick, S. (2010). Monetary Policy in Russia: Identifying exchange rate shocks. Economic Modelling, 27(1), 432-444. https://doi.org/10.1016/j.econmod.2009.10.010
- Guerrieri, V., Lorenzoni, G., Straub, L., & Werning, I. (2020). Macroeconomic Implications of COVID-19: Can Negative Supply Shocks Cause Demand Shortages?. NBER Working Paper, 26918. https://doi.org/10.3386/w26918
- Hansson, J., Jansson, P., & Löf, M. (2003). Business survey data: Do they help forecasting the macro economy (4th Eurostat and DG ECFIN colloquium on modern tools for business cycle analysis). Retrieved from https://ec.europa.eu/eurostat/documents/3888793/5828897/KS-AN-03-051-EN.PDF/aed6487a-4816-4846-9abb-f6cbfd98afd5 on July 24, 2020.
- Hodrick, R.J., & Prescott, E.C. (1997). Postwar U.S. Business Cycles: an Empirical Investigation. Journal of Money Credit and Banking, 29(1), 1-16. Retrieved from https://www.researchgate.net/publication/303087255_Post-war_US_business_cycles_An_empirical_investigation on July 24, 2020.
- International Monetary Fund (2020). World Economic Outlook, October 2020: A Long and Difficult Ascent. Retrieved from https://www.imf.org/en/Publications/WEO/Issues/2020/09/30/world-economic-outlook-october-2020 on November 25, 2020.
- Jorda, O., Singh, S.R., & Taylor, A.M. (2020). Longer-Run Economic Consequences of Pandemics. NBER Work-ing Paper, w26934. https://doi.org/10.3386/w26934
- Kitrar, L., Lipkind, T., & Ostapkovich, G. (2020). Information content of Russian services surveys. Journal of Business Cycle Research, 16(1), 59-74. https://doi.org/10.1007/s41549-020-00040-4
- Kitrar, L., Lipkind, T., Lola, I., Ostapkovich, G., & Chusovlyanov, D. (2015). The HSE ESI and Short-Term Cycles in the Russian Economy. Papers and Studies of Research Institute for Economic Development SGH, 97, 45-66.
- Kitrar, L., & Nilsson, R. (2003). Business Cycles and Cyclical Indicators in Russia. Paris: OECD.
- Korhonen, I., & Mehrotra, A. (2009). Real exchange rate, output and oil: Case of four large energy producers (BOFIT Discussion Paper, 6). https://doi.org/10.2139/ssrn.1428238
- Korhonen, I., & Mehrotra, A. (2010). Money demand in post-crisis Russia: de-dollarisation and remonetisa-tion. Emerging Markets Finance and Trade, 46(2), 5-19. https://doi.org/10.2753/ree1540-496x460201
- Lehmann, R., & Wohlrabe, K. (2013). Forecasting GDP at the Regional Level with Many Predictors. CESifo Working Paper Series, 3956.
- Lipkind, T., Kitrar, L., & Ostapkovich, G. (2019). Russian Business Tendency Surveys by HSE and Rosstat. In S. Smirnov, A. Ozyildirim, & P. Picchetti (Eds.), Business Cycles in BRICS (pp. 233-251). Springer.
- Litterman, R. (1986). Forecasting with Bayesian vector autoregressive model – five years of experience. Jour-nal of Business & Economic Statistics, 4(1), 21-36.
- Lütkepohl, H. (2011). Vector Autoregressive Models. In M. Lovric (Ed.) International Encyclopedia of Statistical Science. Berlin, Heidelberg: Springer. https://doi.org/10.1007/978-3-642-04898-2_609
- Mallick, S.K., & Sousa, R.M. (2013). Commodity Prices, Inflationary Pressures, and Monetary Policy: Evidence from BRICS Economies. Open Economies Review, 24(4), 677-694. https://doi.org/10.1007/s11079-012-9261-5
- Mattos, D., Sequeira, A.N., Lobão, W., & Costa Ferreira, P. (2016). Forecasting Brazilian industrial production with the VAR model and SARIMA with smart dummy (Presentation at the CIRET conference, Copenha-gen).
- Mayr, J., & Ulbricht, D. (2007). VAR Model Averaging for Multi-Step Forecasting. Ifo Working Paper, 48. Re-trieved from https://www.ifo.de/DocDL/IfoWorkingPaper-48.pdf on August 14, 2020.
- Mehrotra, A., & Ponomarenko, A. (2010). Wealth effects and Russian money demand. BOFIT Discussion Pa-per Series, 13. Retrieved from https://doi.org/10.2139/ssrn.1665039 on August 1, 2020.
- Nilsson, R., & Gyomai, G. (2011). Cycle Extraction. A Comparison of the PAT Method, the Hodrick-Prescott and Christiano-Fitzgerald Filters. OECD Statistics Directorate Working Paper, 39. Retrieved from http://www.oecd.org/std/leading-indicators/41520591.pdf on July 28, 2020.
- OECD (2012). OECD System of Composite Leading Indicators. Retrieved from http://www.oecd.org/sdd/leading-indicators/41629509.pdf on November 30, 2020.
- Ollivaud, P., Pionnier, P-A., Rusticelli, E., Schwellnus, C., & Seung-Hee, K. (2016). Forecasting GDP during and after the Great Recession: A contest between small-scale bridge and large-scale dynamic factor model. OECD Economics Department Working paper 1313. Retrieved from https://www.oecd.org/officialdocuments/publicdisplaydocumentpdf/?cote=ECO/WKP(2016)37&docLanguage=En on August 1, 2020.
- Polbin, A. (2020). Ocenka traektorii tempov trendovogo rosta VVP Rossii v ARX-modeli s cenami na neft' Estimating Time-Varying Long-Run Growth Rate of Russian GDP in the ARX Model with Oil Prices. Ekonomicheskaya Politika Economic Policy, 15(1), 40-63. https://doi.org: 10.18288/1994-5124-2020-1-40-63 (In Russian).
- Rautava, J. (2013). Oil prices, Excess Uncertainty and Trend Growth: a Forecasting Model for Russia’s Economy (Austrian Central Bank, Focus on European Economic Integration, 4, pp. 77-87). Retrieved from https://www.oenb.at/dam/jcr:aeee8d5b-ff32-4db7-919c-7e37e5d1fcb9/feei_2013_q4_studies_rautava.pdf on August 14, 2020.
- Rayskaya, N., Sergienko, Ya., Frenkel, A., & Matveeva, O. (2012). Indikator ekonomiki Economy indicator. Ekonomicheskie strategii Economic Strategies, 9, 32-39 (in Russian).
- Sinelnikov-Murylev, S., Drobyshevsky, S., Kazakova, M., & Alexeev, M. (2015). Dekompoziciya tempov rosta VVP Rossii Decomposition of Russia's GDP Growth Rates. Gaidar Institute for Economic Policy. Nauchnye Trudy, 167P (in Russian).
- Smirnov, S. (2020). Predskazanie povorotnykh tochek rossiyskogo ekonomicheskogo tsikla s pomoshch'yu svodnykh operezhayushchikh indeksov Turning Points of the Russian Economic Cycle Using Composite Leading Indicators, Voprosy Statistiki, 27(4), 53-65. https://doi.org/10.34023/2313-6383-2020-27-4-53-65 (in Russian).
- UNECE (2019). Guidelines on Producing Leading, Composite and Sentiment Indicators. Geneva: United Na-tions.