Revolution 4.0 and its implications for consumer behaviour
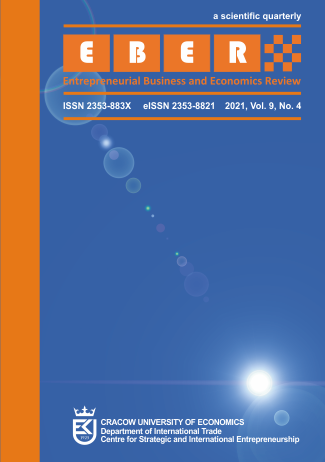
DOI:
https://doi.org/10.15678/EBER.2021.090412Abstract
Objective: The authors attempted to analyze how the achievements of Revolution 4.0 enable the modification of consumers’ decision-making process by means of profiling algorithms. The authors indicate their potential impact, manifested, for example, in micro-targeting, price discrimination and filter bubbles and conclude with a description of radicalization of views.
Research Design & Methods: The paper represents a theoretical study supported by reference to empirical data, the purpose of which is to determine the scale of the impact of IT sector companies on the economy.
Findings: IT tools, available mainly thanks to the achievements of revolution 4.0, allow to modify the content of information at the level of an individual consumer, thus determining their decision-making process. This may entail a new phenomenon of the individualization of mass consumption.
Implications & Recommendations: The benefits of profiling algorithms largely contribute to the improvement of market efficiencies. Nevertheless, the threats accompanying them should be clearly articulated and noticed by policy makers. High-tech enterprises, on the other hand, should assume responsibility for the fair and transparent use of profiling algorithms. Consumers' awareness of the use of this technology should also be raised.
Contribution & Value Added: The article constitutes an original analysis of the approach related to the history of economic theory and the analysis of the most recent events and developments of the current fourth industrial revolution. It examines and shows a holistic approach to the concept of "rationality" from the beginnings of economics as a science until today, along with factors influencing its perception.
Keywords
revolution 4.0, digital revolution, 4th industrial revolution, rationality, consumer behaviour
Author Biography
Wojciech Giza
Associate Professor at the Department of Microeconomics at the Cracow University of Economics (Poland). His scientific research interests include the development of economics as a science and the analysis of theoretical and methodological foundations of neoclassical economics, especially the issues of market failure. As far as institutional economics is concerned, he conducts research on comparative analysis of economic systems with particular emphasis on ordoliberalism and social market economy.
Barbara Wilk
PhD Candidate at the Cracow University of Economics with the specialisation in economics of technological change. Working at the Kosciuszko Institute, Polish non-governmental think tank and research institute, since 2017. She is currently Chief Editor of the European Cybersecurity Journal. She graduated in International Economics and European Studies at the Cracow University of Economics and French Language and Literature at the Jagiellonian University. Her research interests include digital transformation, blockchain technology and impact of artificial intelligence on the labour market.
References
- Babbage, C. (1837[1973]). On the Mathematical Powers of the Calculating Engine. In B. Randell (Ed.), The Origins of Digital Computers, Springer-Verlag Berlin.
- Babbage, C. (1832[2009]). On the Economy of Machinery and Manufactures. New York: Cambridge University Press.
- Borgesius, Z. (2015). Online Price Discrimination and Data Protection Law. Working Paper for the Amsterdam Privacy Conference 2015, Institute for Information Law Research Paper No. 2015-02.
- Bromley, A.G. (1982). Charles Babbage’s Analytical Engine, 1838. Annals of the History of Computing, 4(3), 196-217.
- Dasgupta, S. (2014). It Began with Babbage: The Genesis of Computer Science. Oxford [England]: Oxford Uni-versity Press.
- Ezrachi, A., & Stucke, M. (2016). The rise of behavioral discrimination. European Competition Law Review, 37(12), 485-492. http://dx.doi.org/10.2139/ssrn.2830206
- Fontanel, & J., Sushcheva, N. (2019). La puissance des GAFAM: réalités, apports et dangers. Annuaire français de relations internationales, Paris: La Documentation française, volume XX, 198-222.
- Green, C.D. (2005). Was Babbage’ Analytical Engine Intended to be a Mechanical Model of the Mind? Histo-ry of Psychology, 8(1), 35-45. https://doi.org/10.1037/1093-4510.8.1.35
- Kotlar, J. & Sieger, P. (2018). Bounded Rationality and Bounded Reliability: A Study of Nonfamily Managers’ Entrepreneurial Behavior in Family Firms. Entrepreneurship Theory and Practice, 43(1), 251-273. https://doi.org/10.1177/1042258718796085
- Křenková, E., Rieser, K., & Sato, A. (2021). How software robots can facilitate the procurement process? A case study of Siemens in the Czech Republic. Entrepreneurial Business and Economics Review, 9(3), 191-203. https://doi.org/10.15678/EBER.2021.09031
- Mazurek, G. (2006). Rynek internetowy a uwarunkowania rynku doskonale konkurencyjnego, In G. Sobczyk (Ed.) Zachowania podmiotów na rynku w warunkach konkurencji. Aspekty Marketingowe. Lublin: UMCS.
- Moore, G. (1965). Cramming More Components onto Integrated Circuits. Electronics, 38(8), 114-117.
- Moore, G. (1975). Progress in Digital Integrated Electronics. IEEE Solid-State Circuits Society Newsletter, 11(3), 36-37.
- Ozgur, M.E. (2014). Babbage’s Legacy: The Origins of Microeconomics in “On the Economy of Machinery and Manufactures”. Scottish Journal of Political Economy, 61(3), 322-339. https://doi.org/10.1111/sjpe.12047
- Ricardo, D. (2001 [1821]). On the Principles of Political Economy and Taxation. Kitchener, Ontario: Batoche Books.
- Roncaglia, A. (2019). The Age of Fragmentation. A History of Contemporary Economic Thought. Cambridge UK: Cambridge University Press.
- Rymarczyk, J. (2020). Technologies, Opportunities and Challenges of the Industrial Revolution 4.0: Theoretical Considerations. Entrepreneurial Business and Economics Review, 8(1), 185-198. https://doi.org/10.15678/EBER.2020.080110
- Schwab, K. (2017). The Fourth Industrial Revolution. New York: Penguin Random House.
- Sieja, M., & Wach, K. (2019). The Use of Evolutionary Algorithms for Optimization in the Modern Entrepre-neurial Economy: Interdisciplinary Perspective. Entrepreneurial Business and Economics Review, 7(4), 117-130. https://doi.org/10.15678/EBER.2019.070407
- Simon, H. (1947). Administrative behavior: A Study of Decision‑making Processes in Administrative, New York: The Macmillan Company.
- Simon, H. (1955). A Behavioral Model of Rational Choice, The Quarterly Journal of Economics, 69(1), 99-118. https://doi.org/10.2307/1884852
- Simon, H. (1956). Dynamic Programming Under Uncertainty with a Quadratic Criterion Function, Econometri-ca, 24(1), 74-81. https://doi.org/10.2307/1905261
- Simon, H. (1978). Rationality as Process and as Product of Thought. The American Economic Review, 68(2), 1-16.
- Śledziewska, K., & Włoch, R. (2020). Gospodarka cyfrowa. Jak nowe technologie zmieniają świat. Wydawnic-twa Uniwersytetu Warszawskiego, Warszawa.
- Smith, A. (1795). The Principles which Lead and Direct Philosophical Enquiries; Illustrated by the History of Astronomy. In D. Stewart (Ed.) Essays on Philosophical Subjects. Dublin: The Online Library of Liberty.
- Statista (2020a). Annual net revenue of Amazon, Retrieved from https://www.statista.com/statistics/266282/annual-net-revenue-of-amazoncom/ on 17 June 2020.
- Statista (2020b). Comparison of Apple, Google, Alphabet, and Microsoft from 2008 to 2019, Retrieved from https://www.statista.com/statistics/234529/comparison-of-apple-and-google-revenues/ on 17 June 2020.
- Statista (2020c). Facebook’s annual revenue from 2009 to 2019, Retrieved from https://www.statista.com/statistics/268604/annual-revenue-of-facebook/ on 17 June 2020.
- Statista (2020d). Market value of the largest internet companies worldwide, Retrieved from https://www.statista.com/statistics/277483/market-value-of-the-largest-internet-companies-worldwide/ on 17 June 2020.
- Statista (2020e). Microsoft’s global revenue since 2002, Retrieved from https://www.statista.com/statistics/267805/microsofts-global-revenue-since-2002/ on 17 June 2020.
- Sunstein, C.R. (2014). Choosing Not to Choose. Duke Law Journal, 64(1), 1-52.
- Susskind, R., & Susskind, D. (2015). The future of professions. How technology will transform the work of hu-man experts? Oxford University Press.
- Varian, H. (1989). Price Discrimination. Handbook of Industrial Organization, volume 1, 597-654.
- Verbeke, A. & Greidanus, N.S. (2009). The End of the Opportunism vs Trust Debate: Bounded Reliability as a New Envelope Concept in Research on MNE governance. Journal of International Business Studies, 40(9), 1471-1495.
- Wagner, G., & Eidenmuller, H. (2018). Down by Algorithms? Siphoning Rents, Exploiting Biases, and Shaping Preferences: Regulating the Dark Side of Personalized Transactions. The University of Chicago Law Re-view, 86(2), 581-609.
- Wang Y., & Kosinski M. (2018). Deep neural networks are more accurate than humans at detecting sexual orientation from facial images. Journal of Personality and Social Psychology, 114(2), 246-257. https://doi.org/10.1037/pspa0000098
- Wilkes, M.V. (1977). Babbage as a Computer Pioneer. Historia Mathematica, 4(4), 415-440. https://doi.org/10.1016/0315-0860(77)90079-9